So you’re writing a paper on Generative AI.
You picked this topic because it’s cool, futuristic, and could change the world or steal everyone’s jobs.
But then you fell down the rabbit hole of copyright law, and now your browser history looks like:
“is training AI on copyrighted material illegal,”
“why artists are mad at ChatGPT,”
“fair use AI but for robots”
Been there. And let me tell you, the paper wrote started with this very question:
Should Generative AI Be Allowed to Train on Copyrighted Material Without Consent?
It’s not just philosophical. It’s legal, financial, and deeply human.
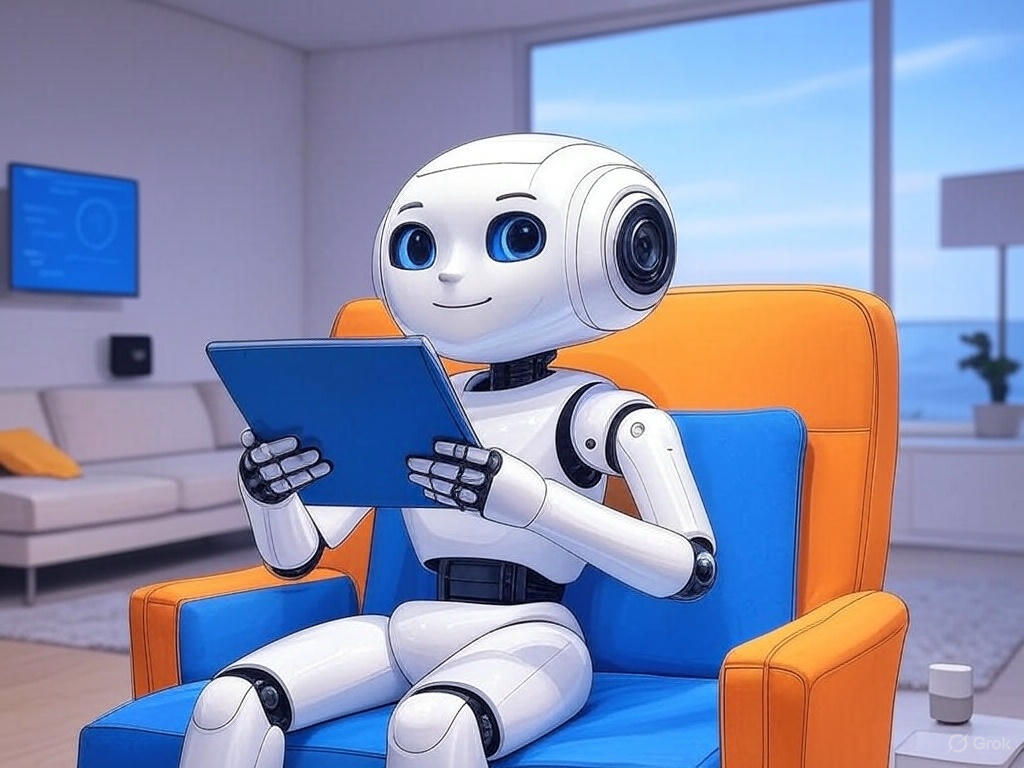
Why This Controversy Is Blowing Up
Here’s the gist:
Generative AI models (like ChatGPT, Midjourney, Stable Diffusion, etc.) are trained on massive datasets, including:
- Online books
- Art portfolios
- News articles
- Reddit threads
- Music lyrics
- Academic journals
Many of these are copyrighted. In most cases, the authors or artists didn’t give consent.
Quick Facts You Can Use in Your Paper:
Topic | Stat or Source |
---|---|
AI models trained on copyrighted data | OpenAI reportedly trained GPT-3 on 300+ billion words, many scraped from books and the web (some behind paywalls) [Wired] |
Authors suing OpenAI | Over a dozen authors, including Sarah Silverman & Paul Tremblay, filed lawsuits in 2023–2024 alleging copyright infringement [NYT] |
Artists vs AI Art Generators | Midjourney and Stability AI face lawsuits for scraping millions of images without permission from platforms like DeviantArt [The Verge] |
Public opinion on AI art use | A 2024 Pew Research survey found 61% of Americans think artists should be compensated when their work is used to train AI |
Legal gray zone | The U.S. Copyright Office stated that current law doesn’t clearly define how training data fits under fair use [CO.gov] |
Your Research Paper Just Got Hot
Forget dry titles like “Applications of AI in Society.”
Your paper could be:
“Neural Theft? The Ethics of Training Generative AI on Copyrighted Work”
“Feed Me Data: Does AI Have a Right to Your Art?”
“Remix or Ripoff? The Legal Black Hole of Generative AI”
And guess what makes your writing even easier (and smarter)? Seamless (seaml.es)
Here’s How I Used Seamless to Slay This Paper (and You Can Too):
1. AI Literature Review That Doesn’t Put You to Sleep
Typed in: “Generative AI copyright lawsuit ethics”
Got:
- Peer-reviewed summaries
- Citation-ready quotes
- Names of the exact lawsuits to reference
Seamless pulls from real academic sources like arXiv and PubMed, not Reddit threads or conspiracy blogs.
2. Podcast Mode
Want to make your research sound cool? Seamless lets you turn your paper into a science podcast in seconds. I made a 10-minute podcast called:
“AI Ate My Novel: Why Authors Are Suing the Machines”
10/10 would recommend blasting this while walking to class feeling like a scholar.
3. Grants and Scholarships
Seamless also showed me grants for researchers and scholarships for computer science students.
Filtered for:
- AI
- Ethics
- Computer Science
- Women in STEM
Found a grant focused on AI ethics worth $15,000.
Drafted Literature Review Sample – took a second and all citations already embedded with links:
Generative AI has been advancing rapidly, prompting considerations about training models on copyrighted material without explicit consent [6]. The ethical and legal implications of this practice are becoming increasingly pertinent, urging researchers and developers to reevaluate their approach [15]. As the release of new Generative AI tools outpaces the establishment of regulatory frameworks, the need for guidance in education and research becomes evident [2]. In the context of generative AI, the balance between innovation and copyright protection is delicate. Recent studies have explored the potential benefits and drawbacks of incorporating copyrighted material into AI training datasets. For instance, adding copyrighted books and newspapers to the data mixture of Large Language Models (LLMs) can influence their performance, highlighting the complex interplay between AI capabilities and copyrighted content [7]. Meanwhile, the impact of watermarking LLMs to prevent the generation of copyrighted text indicates the challenges and trade-offs associated with safeguarding intellectual property in AI systems [9]. Addressing these concerns, researchers have proposed innovative solutions to detect the inclusion of copyrighted content during model development, offering new avenues for ensuring compliance with copyright laws and ethical standards [10]. Additionally, the idea of uniqueness in model training has been suggested as a way to protect copyrighted material, emphasizing the importance of employing unique identifiers for reliable and independent membership inference [6]. As the field of generative AI continues to evolve and expand, the discourse around training models on copyrighted material without consent underscores the necessity for comprehensive guidelines and ethical frameworks [2]. Balancing innovation and respect for intellectual property rights remains a critical challenge that researchers and developers must navigate in this rapidly evolving landscape.
All thanks to Seamless. Now go forth and write the next AI manifesto with proper citations, of course.